Python For Quantum Mechanics#
Week 6: Scipy Optimization#
from IPython.display import YouTubeVideo
YouTubeVideo('p2ohSsd1KTg',width=700, height=400)
import matplotlib.pyplot as plt
import numpy as np
import numpy.random as rnd
import scipy
from scipy import optimize
---------------------------------------------------------------------------
ModuleNotFoundError Traceback (most recent call last)
Cell In[2], line 4
2 import numpy as np
3 import numpy.random as rnd
----> 4 import scipy
5 from scipy import optimize
ModuleNotFoundError: No module named 'scipy'
Function Minimum#
def f(b):
return -(.6*np.log(1+b[0]-b[1]-b[2])+.3*np.log(1-b[0]+2*b[1]-b[2])+.1*np.log(1-b[0]-b[1]+4*b[2]))
from scipy.optimize import Bounds
bounds = Bounds([0.0, 1.0], [0.0, 1.0],[0.0, 1.0])
initial_guess = np.array([0.0,0.0,0.0])
result = optimize.minimize(f, initial_guess, bounds=bounds)
print(result)
---------------------------------------------------------------------------
IndexError Traceback (most recent call last)
Input In [23], in <cell line: 4>()
2 bounds = Bounds([0.0, 1.0], [0.0, 1.0],[0.0, 1.0])
3 initial_guess = np.array([0.0,0.0,0.0])
----> 4 result = optimize.minimize(f, initial_guess, bounds=bounds)
5 print(result)
File /usr/local/lib/python3.9/site-packages/scipy/optimize/_minimize.py:643, in minimize(fun, x0, args, method, jac, hess, hessp, bounds, constraints, tol, callback, options)
638 i_fixed = (bounds.lb == bounds.ub)
640 if np.all(i_fixed):
641 # all the parameters are fixed, a minimizer is not able to do
642 # anything
--> 643 return _optimize_result_for_equal_bounds(
644 fun, bounds, meth, args=args, constraints=constraints
645 )
647 # determine whether finite differences are needed for any grad/jac
648 fd_needed = (not callable(jac))
File /usr/local/lib/python3.9/site-packages/scipy/optimize/_minimize.py:1007, in _optimize_result_for_equal_bounds(fun, bounds, method, args, constraints)
1001 success = False
1002 message = (f"All independent variables were fixed by bounds, but "
1003 f"the independent variables do not satisfy the "
1004 f"constraints exactly. (Maximum violation: {maxcv}).")
1006 return OptimizeResult(
-> 1007 x=x0, fun=fun(x0, *args), success=success, message=message, nfev=1,
1008 njev=0, nhev=0,
1009 )
Input In [10], in f(b)
1 def f(b):
----> 2 return -(.6*np.log(1+b[0]-b[1]-b[2])+.3*np.log(1-b[0]+2*b[1]-b[2])+.1*np.log(1-b[0]-b[1]+4*b[2]))
IndexError: index 2 is out of bounds for axis 0 with size 2
Given some function we are able to find a local minimum using scipy.optimize.fmin(f,x0)
, where f
is the function in question, x0
is an initial guess.
def f(x):
return x**4 + x**3 + x**2 - 7*x + 10
x = np.linspace(-3,3,100)
y = f(x)
fig = plt.figure()
ax = fig.add_axes([.1,.1,.8,.8])
ax.plot(x,y)
plt.show()
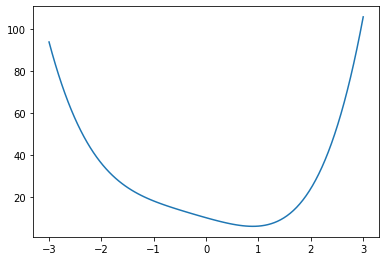
x_min = optimize.fmin(f, 0)
print(x_min)
Optimization terminated successfully.
Current function value: 5.894475
Iterations: 24
Function evaluations: 48
[0.8914375]
However if the function has multiple local minima the initial guess will determine which is found.
def g(x):
return x**4 - x**3 - 5* x**2 + x + 0
x = np.linspace(-2,3,100)
y = g(x)
fig = plt.figure()
ax = fig.add_axes([.1,.1,.8,.8])
ax.plot(x,y)
plt.show()
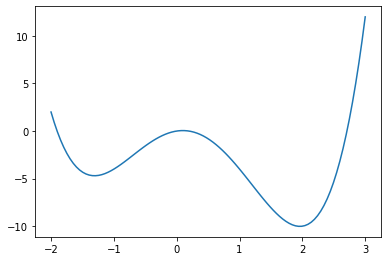
x_min1 = optimize.fmin(g, -1)
print('x_min1 =',x_min1,'\n')
x_min2 = optimize.fmin(g, 1)
print('x_min2 =',x_min2)
Optimization terminated successfully.
Current function value: -4.697455
Iterations: 14
Function evaluations: 28
x_min1 = [-1.3078125]
Optimization terminated successfully.
Current function value: -10.019646
Iterations: 16
Function evaluations: 32
x_min2 = [1.96025391]
Global Optimization#
We can find the global minimum of a function, within given bounds, using scipy.optimize.differential_evolution(func,bounds)
. bounds
will be a list of lists containing the max and min of the input parameters of func
. It should be noted that this works best when func
takes in one argument which is a list of the input parameters. Running this function returns the information about the optomisation process with the min value found stored as x
.
result = scipy.optimize.differential_evolution(g,[[-2,3]])
print(result)
fun: array([-10.01964635])
jac: array([-1.42108548e-06])
message: 'Optimization terminated successfully.'
nfev: 68
nit: 3
success: True
x: array([1.96027333])
A similar process can be achieved with multivariable functions too.
def f(params):
return -100. * np.exp(-0.1*np.sqrt(params[0]**2 + params[1]**2)) + np.exp(np.cos(params[0]) + np.cos(params[1]))
x_bound = [-20,20]
y_bound = [-20,20]
xs = np.linspace(x_bound[0], x_bound[1], 100)
ys = np.linspace(y_bound[0], y_bound[1], 100)
Xmesh,Ymesh = np.meshgrid(xs, ys)
Zmesh = f([Xmesh,Ymesh])
fig = plt.figure(figsize=(7,7))
ax = fig.add_axes([.1,.1,.8,.8],projection='3d')
ax.contour3D(Xmesh,Ymesh,Zmesh,90,cmap='seismic')
plt.show()
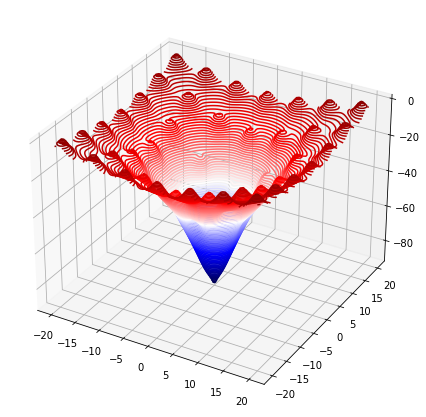
bounds = [x_bound,y_bound]
result = scipy.optimize.differential_evolution(f,bounds)
print(np.round(result.x,2))
[-0. -0.]
Function Roots#
The function scipy.optimize.fsolve(f,x0)
finds the roots of a function f
, given an initial guess array x0
.
randy = rnd.random(3)
def f(x):
return 0.25*randy[0]*x**2 - randy[1]*2*x - randy[2]
x = np.linspace(-20,40,100)
y = f(x)
y2 = np.zeros(len(x))
fig = plt.figure()
ax = fig.add_axes([.1,.1,.8,.8])
ax.set_xlim(x[0],x[-1])
ax.plot(x,y,x,y2)
plt.show()
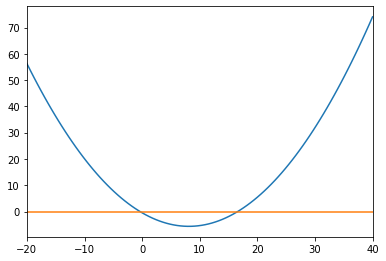
roots = optimize.fsolve(f,[x[0],x[-1]])
print(roots)
print(np.round(f(roots),2))
[-0.37906637 16.59148356]
[-0. 0.]
Optimize Least Square Fit#
Lets try and fit a curve to the following noisy data.
c1, c2 = 0.5, 2.0
x = np.arange(20)
y = c1*x**2 + c2*x
noise = y + 0.2 * np.max(y) * rnd.random(len(y))*((-1)**(2-(np.round(rnd.random(len(y)),0))))
fig = plt.figure()
ax = fig.add_axes([.1,.1,.8,.8])
ax.plot(x,noise,'x',label='Noise')
ax.set_xlabel('x')
ax.set_ylabel('y')
ax.legend()
plt.show()
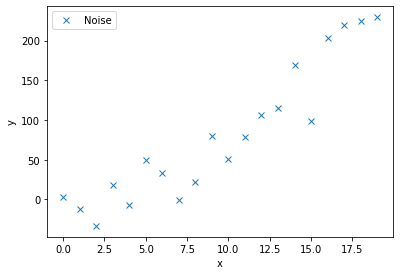
We will now define a function to model this curve, as well as a function that calculates the residuals. The residuals are the differrence between each noisy data point and the model being fitted. This scipy.optimize.leastsq(residuals,c_guess, args=(noise,x))
function gives back the coefficient c
that minimises the residuals. We must input the noisy data, noise
, and their corresponding x
co-ordinates, as well as an intial guess for the coefficients c_guess
.
def model(x, c):
return c[0]*x**2 + c[1]*x
def residuals(c,y,x):
return y - model(x,c)
c_guess = np.array([0.0,2.1])
c_result, flag = scipy.optimize.leastsq(residuals,c_guess, args=(noise,x))
print(c_result)
fig = plt.figure()
ax = fig.add_axes([.1,.1,.8,.8])
ax.plot(x, model(x,c_result), label='Model')
ax.plot(x, noise, 'x', label='Noise')
ax.set_xlabel('x')
ax.set_ylabel('y')
ax.legend()
plt.show()
[ 0.7283107 -0.65686655]
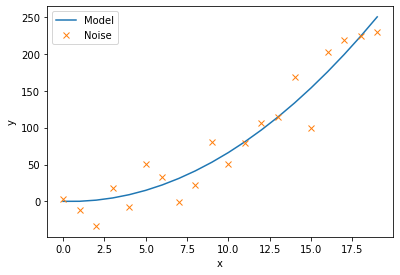
Curve Fit#
The scipy.optimize.curve_fit(model,xdata,ydata,p0)
function allows us to directly input the model
function aswell the noisy data, (xdata
,ydata
), along with an initial guess for the model coefficients p0
. We must define our model function with indiviual coefficeients rather than an array of cefficients.
def model(x, c1, c2):
return c1*x**2 + c2*x
c_result, flag = scipy.optimize.curve_fit(model,x,noise,p0=(0.5,2.0))
print(c_result)
fig = plt.figure()
ax = fig.add_axes([.1,.1,.8,.8])
ax.plot(x,model(x,c_result[0],c_result[1]),label='Model')
ax.plot(x,noise,'x',label='Noise')
ax.set_xlabel('x')
ax.set_ylabel('y')
ax.legend()
plt.show()
[ 0.72831071 -0.65686659]
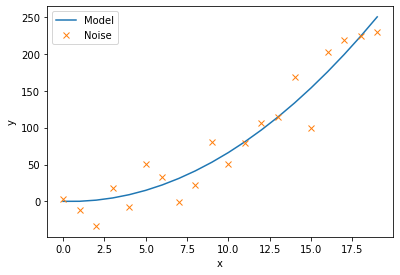